- announcement in the field of neuromorphic computing
- Efficiency and sustainability improvements for AI systems
- Potential for real-time continuous learning and deployment of AI capabilities
Intel, the tech giant, has made a announcement today. They have successfully developed the world’s largest neuromorphic system, codenamed Hala Point. This impressive system, powered by Intel’s Loihi 2 processor, is designed to support research in brain-inspired artificial intelligence (AI) and address the challenges faced by today’s AI systems in terms of efficiency and sustainability. Hala Point represents a significant advancement from Intel’s previous large-scale research system, Pohoiki Springs, with architectural improvements that result in over 10 times more neuron capacity and up to 12 times higher performance.
According to Mike Davies, the director of the Neuromorphic Computing Lab at Intel Labs, the computing cost of current AI models is skyrocketing at an unsustainable rate. To tackle this issue, Intel developed Hala Point, which combines the efficiency of deep learning with innovative brain-inspired learning and optimization capabilities. The hope is that research conducted with Hala Point will enhance the efficiency and adaptability of large-scale AI technology.
So, what exactly does Hala Point do? It is the first large-scale neuromorphic system to demonstrate state-of-the-art computational efficiencies on mainstream AI workloads. It has been characterized as capable of supporting up to 20 quadrillion operations per second, or 20 petaops, with an efficiency exceeding 15 trillion 8-bit operations per second per watt (TOPS/W) when running conventional deep neural networks. These performance levels rival and even surpass those achieved by architectures relying on graphics processing units (GPU) and central processing units (CPU). The unique capabilities of Hala Point have the potential to enable real-time continuous learning for various AI applications, including scientific problem-solving, logistics, smart city infrastructure management, large language models (LLMs), and AI agents.
Sandia National Laboratories, for their advanced research endeavors, will be utilizing Hala Point for brain-scale computing research. Their focus will be on solving scientific computing problems in fields such as device physics, computer architecture, computer science, and informatics. Craig Vineyard, the Hala Point team lead at Sandia National Laboratories, expressed excitement about the enhanced capabilities that Hala Point brings to their team, enabling them to keep up with the evolution of AI across various sectors.
It is important to note that Hala Point is currently a research prototype, but Intel anticipates that the lessons learned from its development will pave the way for practical advancements in future commercial systems. For example, continuous learning for large language models (LLMs) could become a reality, significantly reducing the training burden associated with widespread AI deployments.
The significance of Hala Point lies in the recent challenges faced by AI in terms of scaling up deep learning models to trillions of parameters. These challenges have highlighted the need for innovation at the hardware architecture level. Neuromorphic computing, a novel approach drawing inspiration from neuroscience, integrates memory and computing with highly parallelized operations to minimize data movement. In fact, Loihi 2, the processor powering Hala Point, has already demonstrated remarkable gains in efficiency, speed, and adaptability when applied to small-scale edge workloads.
Building upon the foundation laid by its predecessor, Pohoiki Springs, Hala Point brings neuromorphic performance and efficiency gains to mainstream deep learning models. This is particularly beneficial for real-time workloads such as video processing, speech recognition, and wireless communications. For instance, Ericsson Research is already leveraging Loihi 2 to optimize telecom infrastructure efficiency.
Let’s delve into the technical specifications of Hala Point. It comprises 1,152 Loihi 2 neuromorphic processors produced on Intel 4 process node, housed in a six-rack-unit data center chassis about the size of a microwave oven. The system supports a staggering 1.15 billion neurons and 128 billion synapses, distributed across 140,544 neuromorphic processing cores. It consumes a maximum of 2,600 watts of power and includes over 2,300 embedded x86 processors for additional computations.
Hala Point integrates processing, memory, and communication channels in a massively parallelized fabric, providing an impressive total of 16 petabytes per second (PB/s) of memory bandwidth, 3.5 PB/s of inter-core communication bandwidth, and 5 terabytes per second (TB/s) of inter-chip communication bandwidth. The system is capable of processing over 380 trillion 8-bit synapses and over 240 trillion neuron operations per second.
When applied to bio-inspired spiking neural network models, Hala Point can execute its full capacity of 1.15 billion neurons up to 20 times faster than the human brain. Even at lower capacities, it can achieve speeds up to 200 times faster. Although Hala Point is not designed for neuroscience modeling specifically, its neuron capacity is roughly equivalent to that of an owl brain or the cortex of a capuchin monkey.
The energy efficiency and performance gains offered by Loihi-based systems are truly remarkable. They can perform AI inference and solve optimization problems using 100 times less energy while operating up to 50 times faster than conventional CPU and GPU architectures. By leveraging sparse connectivity and event-driven activity up to a ratio of 10:1, early results from Hala Point demonstrate deep neural network efficiencies as high as 15 TOPS/W2. Moreover, unlike GPUs that require input data to be collected into batches, Hala Point can process real-time data, such as video feeds, without significant delays.
While Hala Point is currently a research prototype, its deployment at Sandia National Laboratories marks the beginning of Intel’s plan to share this new family of large-scale neuromorphic research systems with its collaborators. The further development of this technology will enable neuromorphic computing applications to overcome power and Latency constraints, ultimately allowing for real-world, real-time deployment of AI capabilities.
Intel is working closely with its Intel Neuromorphic Research Community (INRC), consisting of over 200 members, including leading academic groups, government labs, research institutions, and companies worldwide. Together, they aim to push the boundaries of brain-inspired AI and transition this technology from research prototypes to industry-leading commercial products in the coming years.
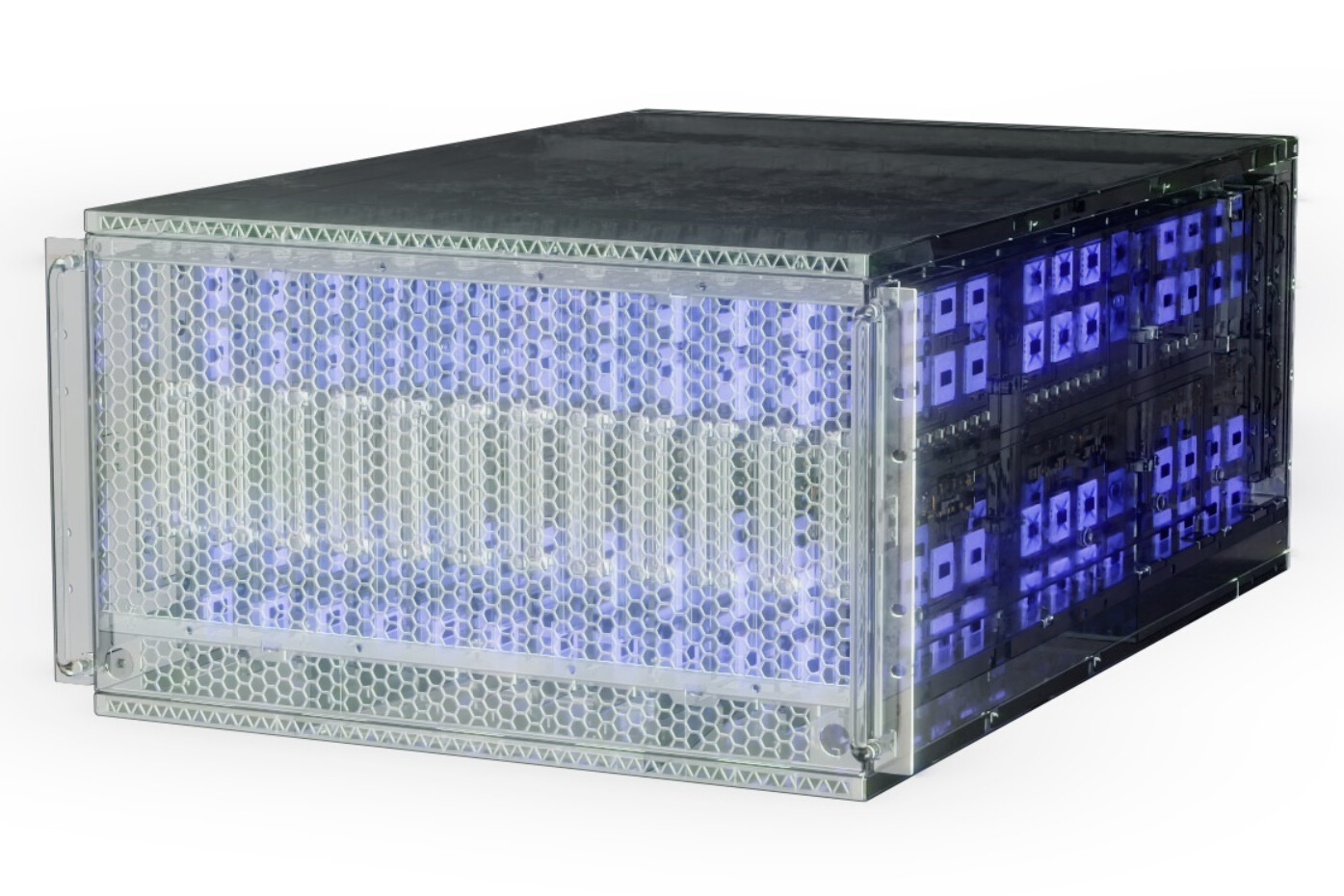
About Our Team
Our team comprises industry insiders with extensive experience in computers, semiconductors, games, and consumer electronics. With decades of collective experience, we’re committed to delivering timely, accurate, and engaging news content to our readers.
Background Information
About Intel: Intel Corporation, a global technology leader, is for its semiconductor innovations that power computing and communication devices worldwide. As a pioneer in microprocessor technology, Intel has left an indelible mark on the evolution of computing with its processors that drive everything from PCs to data centers and beyond. With a history of advancements, Intel's relentless pursuit of innovation continues to shape the digital landscape, offering solutions that empower businesses and individuals to achieve new levels of productivity and connectivity.
Technology Explained
CPU: The Central Processing Unit (CPU) is the brain of a computer, responsible for executing instructions and performing calculations. It is the most important component of a computer system, as it is responsible for controlling all other components. CPUs are used in a wide range of applications, from desktop computers to mobile devices, gaming consoles, and even supercomputers. CPUs are used to process data, execute instructions, and control the flow of information within a computer system. They are also used to control the input and output of data, as well as to store and retrieve data from memory. CPUs are essential for the functioning of any computer system, and their applications in the computer industry are vast.
GPU: GPU stands for Graphics Processing Unit and is a specialized type of processor designed to handle graphics-intensive tasks. It is used in the computer industry to render images, videos, and 3D graphics. GPUs are used in gaming consoles, PCs, and mobile devices to provide a smooth and immersive gaming experience. They are also used in the medical field to create 3D models of organs and tissues, and in the automotive industry to create virtual prototypes of cars. GPUs are also used in the field of artificial intelligence to process large amounts of data and create complex models. GPUs are becoming increasingly important in the computer industry as they are able to process large amounts of data quickly and efficiently.
Latency: Technology latency is the time it takes for a computer system to respond to a request. It is an important factor in the performance of computer systems, as it affects the speed and efficiency of data processing. In the computer industry, latency is a major factor in the performance of computer networks, storage systems, and other computer systems. Low latency is essential for applications that require fast response times, such as online gaming, streaming media, and real-time data processing. High latency can cause delays in data processing, resulting in slow response times and poor performance. To reduce latency, computer systems use various techniques such as caching, load balancing, and parallel processing. By reducing latency, computer systems can provide faster response times and improved performance.
Trending Posts
NVIDIA GeForce NOW adds Men of War II, Palworld, and other games this week.
Palit introduces Stylish White Series: GeForce RTX 4070 and RTX 4060 Ti
Western Digital Reveals Record-breaking 2.5″ Portable HDDs with Unmatched Storage Capacity
Sabrent introduces DS-UICA USB-C docking station for smartphones and ultraportables, a game-changer.
ASUS introduces Infinite Wealth Game Bundle for NVIDIA and AMD Graphics Cards
Evergreen Posts
NZXT about to launch the H6 Flow RGB, a HYTE Y60’ish Mid tower case
Intel’s CPU Roadmap: 15th Gen Arrow Lake Arriving Q4 2024, Panther Lake and Nova Lake Follow
HYTE teases the “HYTE Y70 Touch” case with large touch screen
NVIDIA’s Data-Center Roadmap Reveals GB200 and GX200 GPUs for 2024-2025
Intel introduces 2023-2025 CPU Roadmap: 15th Gen Arrow Lake, Panther, and Nova Lake Revealed